Introduction
Prelude
Once upon a time in the world of artificial intelligence, where the only things faster than the latest quantum computing breakthroughs were the governments’ attempts to ‘watermark’ the outputs of AI. If this isn’t a classic sci-fi twist, then we don’t know what is! But never fear, dear reader, for we are here to dissect this digital drama for you, sprinkle in a dash of decentralized reasoning, and offer a side dish of potential solutions. Hold onto your transistors, because it’s going to be a wild ride!
Our story begins in a world where the power of AI has evolved at a breakneck speed, throwing traditional governance and control into a frenzy. To keep pace, our esteemed governments have floated the idea of ‘watermarking’ AI outputs. Imagine that – every witty one-liner and profound sentiment served up by your friendly neighborhood AI could soon come with its own digital birthmark. While this may sound like a great plot for a dystopian novel, the reality could be far from fiction.
Meanwhile, in another part of the tech universe, quantum computing advancements are shifting the very foundation of computation. China’s Jiuzhang computer is now proudly strutting about, claiming to perform tasks commonly used in AI a whopping 180 million times faster than the world’s most powerful supercomputer. It’s like your ordinary computer just gulped down an entire gallon of superhero potion! But amidst all these thrilling developments, how can we find a common denominator? Well, we believe that ‘decentralization’ could be the linchpin that holds everything together. Picture this – a world where AI is decentralized, giving everyone a fair shot at harnessing its power. A world where the guardianship of AI is not in the hands of a few tech giants, but instead, governed through a decentralized voting mechanism. Sounds like a tech utopia, doesn’t it?
Here is where we introduce GRIDNET OS – a valiant protagonist in our tale. It could be the chosen platform to host the decentralized AI of the future. Before you question, “why GRIDNET OS?”, we promise to answer this question in the unfolding chapters of our digital saga. So, buckle up, dear reader! We’re about to embark on a journey through the roller-coaster world of AI, quantum computing, and decentralization. And remember, no matter how fast or high this ride goes, there’s no need to fear, for we’re all in this together – each one of us part of this exciting tale of the future of AI. Enjoy the ride!
Watermarks, but does water go along well with AI?
Is the concept of watermarking AI created content even valid? Isn’t it like an attempt of watermarking water ? Or air? In the rapidly advancing landscape of Artificial Intelligence (AI), the task of regulating AI-generated content poses significant challenges. As AI continues to produce increasingly realistic and convincing content, from innocuous deepfakes to potentially harmful disinformation campaigns, governments and regulatory bodies worldwide are grappling with the necessity to curb misuse. The Chinese government, among others, has implemented mandatory watermarking of AI-generated content as a regulation strategy. While the aim is to provide transparency and traceability, this approach has several inherent issues and potential negative impacts.
AI watermarking, at its core, involves embedding distinct markers in AI-generated content to identify them as such. Although watermarking has proven effective for image-based content, it presents complex challenges when applied to text-based AI outputs. The restrictions imposed by the semantics and syntax of language make the process of subtly altering text to embed watermarks particularly tricky (were these to contain any information in regard to ‘prompts’) , and can potentially affect the underlying meaning of the content. Peculiarities as these aside many of us who use AI generated art for articles and materials for a variety of purposes simply do not want their contents to be ‘flagged’ in the eyes of the recipient as being of a lower quality or whatever. We simply do not need that. Same as we do not want clothes which have been washed in a washing machine to wear a sticker of not having been washed manually.
Moreover, this approach raises significant concerns about the enforceability of regulations, the potential for misuse of watermarks, and the risk of real harms arising from false positives during watermark detection. For instance, innocent individuals could be wrongfully accused of misconduct if their human-generated content is falsely flagged as AI-generated. Furthermore, adversaries could maliciously mimic watermarks to deceive detection systems or to create false trails, thus compounding the issue of disinformation.
In light of these challenges, it becomes increasingly evident that we are in urgent need of alternative solutions that maintain the progress of AI without imposing restrictive regulations that may inadvertently hinder innovation or lead to misuse. In this article, we propose an innovative approach: leveraging the power of decentralized open AI algorithms to ensure unhindered progress in AI.
Importance and need for an alternative approach: Decentralized open AI algorithms.
To address the limitations and potential adverse effects of AI watermarking regulations, we advocate for an alternative approach – the utilization of decentralized open AI algorithms. This approach, with its roots in blockchain technology and decentralized architecture, could provide a promising alternative to the traditional centralized AI systems.
Centralized AI systems concentrate data and control in one place or entity, which makes them susceptible to misuse, security breaches, and single points of failure. Moreover, such centralized structures tend to stifle innovation, as they restrict the access and contribution to AI algorithms and models to a select few. Conversely, decentralized AI systems distribute data and control across multiple nodes, increasing resilience, security, and fostering a more inclusive, collaborative environment for innovation. On the other hand, decentralized open AI algorithms could be inherently transparent, promoting trust and accountability. Each of the network could use her voting power to flag a given piece of art, making it difficult for harmful actions or manipulations to go unnoticed. This transparency stands in contrast to the oblique nature of watermarking, which can potentially be manipulated or misused.
By leaning towards decentralized open AI algorithms, we would encourage a more dynamic and adaptable AI landscape. Unlike a singular algorithm in centralized services, a decentralized approach could enable for establishment of diverse sub-grids or ‘realms’, each featuring distinct characteristics and evolving according to user feedback and reinforcement learning. This flexibility could allow AI algorithms to cater more accurately to specific users’ expectations, fostering a more personalized and efficient AI experience. The importance of this alternative approach is even more pronounced in the face of burgeoning AI capabilities. As AI-generated content becomes increasingly prevalent and indistinguishable from human-generated content, it is imperative to have a robust, transparent, and adaptable framework that supports the positive growth of AI while effectively mitigating its potential risks. The decentralized open AI algorithms offer a viable solution to this challenge. Through voting and flagging, instead of watermarking.
Brief description of the proposed solution: Utilizing GRIDNET OS for decentralizing AI, with a special emphasis on the decentralized stable diffusion process.
Remember the old cartoon about the Jetsons, where AI is seamlessly integrated into daily life, from Rosie the robot maid to George Jetson’s flying car? Well, we’re not there yet, and we don’t have flying cars or a robot named Rosie, but we have something arguably even cooler: the GRIDNET Decentralized Operating System (GRIDNET OS).
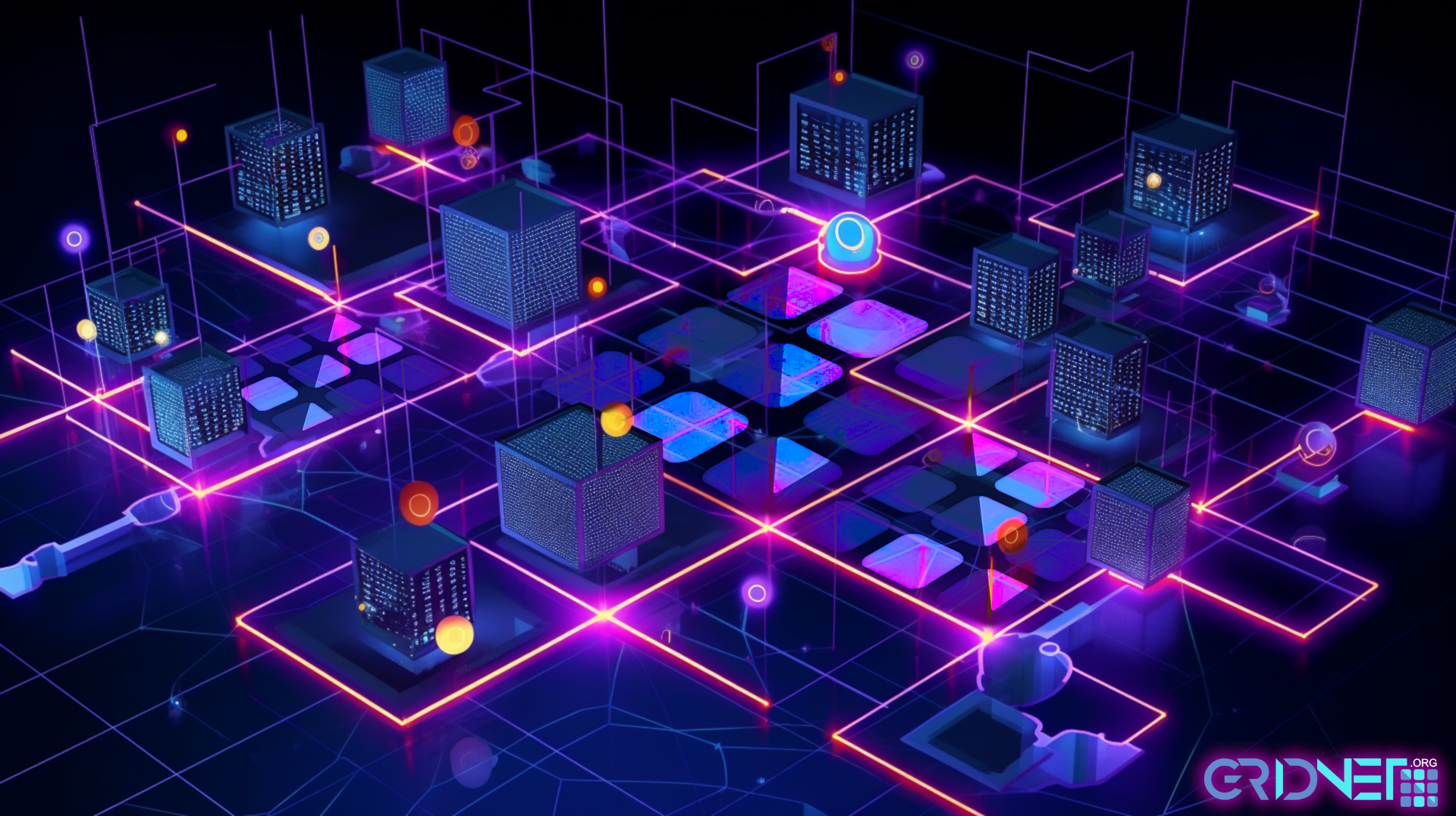
Decentralization, meaning evolution seemingly always finds a way..
Before you yawn and click away, hear us out. This isn’t just another jargon-filled, techy mumbo-jumbo about a software system that only coders can comprehend. Picture this: a platform that’s got more multi-functionality than a Swiss army knife, only instead of a corkscrew and a pair of scissors, it’s got the key to transform how we handle AI. Curious yet?
As a suitable platform for implementing our proposed approach, we bring forth the GRIDNET Decentralized Operating System (GRIDNET OS). It’s a comprehensive and efficient system that supports a diverse range of functionalities essential for creating and managing decentralized open AI algorithms.
GRIDNET OS offers several key features that make it an excellent candidate for hosting the mechanics of decentralized AI. These include
- Decentralized User-Interface Mechanics: GRIDNET OS supports decentralized user-interface mechanics, enabling a distributed, user-driven approach to interaction with AI-generated content. Like, it interacting with AI would need to boil down to Discord and web-chat no more.
- Decentralized Storage with Arbitrary Redundancy Guarantees: It offers a robust and resilient data storage solution that allows for data redundancy across various nodes in the network, thereby enhancing data security and accessibility.
- Extremely Efficient Off-The-Chain Transactions (TXs): The system’s ability to perform off-the-chain transactions facilitates swift, efficient transactions without compromising the integrity of the blockchain.
- Decentralized Computing: GRIDNET OS allows for decentralized computing, distributing computational tasks across various nodes, thereby increasing the system’s scalability and performance (GPUs)
- Decentralized File-System with Decentralized Access Rights’ Provisioning: This unique feature of GRIDNET OS allows for controlled access to information in a decentralized manner, protecting user data while ensuring the necessary accessibility (decentralized access rights’ provisioning)
- Built-In Decentralized Voting Constructs within Autonomous Enforcement of Polls’ Results: This feature provides a democratic and decentralized method of decision-making within the system, empowering users to have a say in significant decisions, such as moderating the network or altering the algorithms
- Decentralized Identity with an Inherent Concept of a Stake: GRIDNET OS also features a system of decentralized identities, enhancing user privacy and control over personal data.
We will be seeing into how these functionalities could benefit decentralized AI later on. To keep you excited just fathom that implementation of decentralized stable diffusion processes within GRIDNET OS would employ reinforcement learning and user feedback to continuously enhance the algorithms in a distributed manner. Nodes generating art or other AI-driven content through stable diffusion algorithms would be rewarded with the system-intrinsic cryptocurrency, thereby promoting active participation and innovation. Such a decentralized approach would ensure continuous, unhindered progress of AI while maintaining a high degree of accountability and control.
Section 1: Current State of AI Regulation
A review of the current situation and problems with watermarking for text-based content.
AI-generated content is becoming increasingly pervasive in our everyday lives. However, its rapidly advancing capabilities are being accompanied by new concerns over its potential misuse. Recently, this has prompted some countries, such as China, to implement regulations for labeling and watermarking AI-generated content. Despite the good intentions, the application of watermarking, especially for text-based AI-generated content, poses unique challenges that necessitate a closer examination. The use of watermarks has a long-standing history in content tracking and violation detection of intellectual property rights. Traditional image-based watermarking involves adding barely perceptible changes to an image that can serve as a unique identifier. However, a similar strategy for text-based content presents significant challenges, to let others know that it’s not ‘real’ is a whole a different kind of a story.
Two approaches to watermarking of AI content
In the broad landscape of AI watermarking, we can differentiate between two distinctive approaches: Proactive Watermarking and Reactive Watermarking.
Proactive Watermarking is an approach where the watermark is introduced during the content generation process by the service provider or the company itself. This method allows for the direct integration of the watermark into the AI algorithm’s output, giving the generating company control over the watermarking process. Such an approach ensures that every piece of content produced carries an identifiable marker right from its inception. However, this method hinges upon the transparency and willingness of the AI service provider to embed watermarks into their content. Service provider might be very reluctant as understandably this renders products of their AI services as of ‘lower-quality’ in the eyes of the recipient. And ‘centralized’ AI service providers want to have a paying client base.
On the other hand, Reactive Watermarking is the process where an external algorithm, usually owned and managed by a regulatory or enforcement body, would presumably be used to insert watermarks into AI-generated content after its creation. This approach may be necessary in scenarios where the enforcement body does not have direct control over the AI algorithm or when the AI service provider may be unwilling or unable to include watermarks during content generation. Despite being a reactive measure, this approach allows for post-hoc tracing of AI-generated content and ensures that content can be marked even if it was initially produced without a watermark. This approach however has an extremely large surface for producing false positives harming legitimate art and content producers.
Each of these approaches carries its advantages and challenges. Proactive watermarking requires cooperation from AI service providers, but ensures comprehensive coverage, while reactive watermarking allows for regulatory intervention, albeit at the risk of missing content that circulates quickly or goes under the radar or in the worst case (but very likely scenario) producing false-positives. Understanding these differences is crucial when designing systems and regulations for the governance of AI-generated content. For instance, if an academic paper is incorrectly flagged as AI-generated content, the student could be unjustly accused of academic misconduct, affecting their academic and professional future. Similarly, non-native English writing samples are at a higher risk of being misclassified as AI-generated, which could lead to potential bias and discrimination. Therefore, while the concept of watermarking AI-generated content appears to be a viable solution at face value, its practical application, especially for text-based content, is riddled with challenges that cannot be overlooked. Policymakers must therefore carefully consider these factors before proceeding with regulations centered around watermarking.
Challenges of implementing AI watermark regulations in the United States or the European Union.
In contemplating the application of AI watermarking regulations, policymakers in the United States or the European Union are faced with an intricate tapestry of challenges. These issues range from enforcement, to potential misuse, to reconciling with freedom of speech.
In principle, a regulatory requirement mandating companies to label or watermark AI-generated content could be beneficial. Watermarking could facilitate downstream research, potentially helping to identify the prevalence and origin of AI-generated disinformation. For instance, one could measure how content from a specific service spreads through internet platforms, factoring in the accuracy of watermark detection mechanisms. However, enforceability of such regulations presents a significant hurdle. The imperfections of reactive text-based watermarking systems are likely to result in instances where human-generated content is incorrectly flagged as AI-generated. This risk of false positives can have real-world implications. For example, students might be unjustly accused of academic misconduct, impacting their educational trajectory, if their work is incorrectly flagged as AI-generated.
Further, these imperfections can also be exploited. Adversaries could purposefully mimic a watermark, creating a misleading trail of AI-generated content for nefarious purposes. This presents a threat to the integrity of information and the safety of online spaces. The regulations requiring users not to alter or remove watermarks can prove problematic. Given the challenges associated with watermarking text-based content, how can one reliably determine that a text-based watermark was removed? In a country like the United States, with strong protections for freedom of speech, regulations restricting individual expression could face significant constitutional challenges.
The feasibility of prominently labeling AI-generated content might seem like a simpler solution. However, the process becomes complicated when dealing with user-contributed content of unknown provenance. Would the individual user be held liable for uploading AI-generated content without a label? Or would the platform be held accountable? To determine this, the same problematic AI detectors would be necessary, resulting in the aforementioned challenges. Given these uncertainties and complexities, the United States and the European Union need to tread carefully in contemplating watermarking legislation similar to China’s. The risks associated with watermarking must be considered alongside the potential benefits. Indeed, while the current conversation revolves around the applicability of watermarking for AI content regulation, perhaps it also signals the need for exploring alternative approaches to address the challenges posed by AI-generated content.
The Future
Now, imagine a world where AI isn’t just the tool of large tech companies but is truly decentralized, with countless algorithms creating content from servers scattered across the globe. In this world, Johnny, an amateur poet, uses an AI poetry generator hosted in Singapore from his home in Belgium to create beautiful verses that are indistinguishable from human-written ones. He then posts these poems on an American social media platform, enjoyed by his followers who hail from countries around the world. But wait a sec.. the service provider in Singapore is just a node within of a decentralized network.. just like in Torrent.
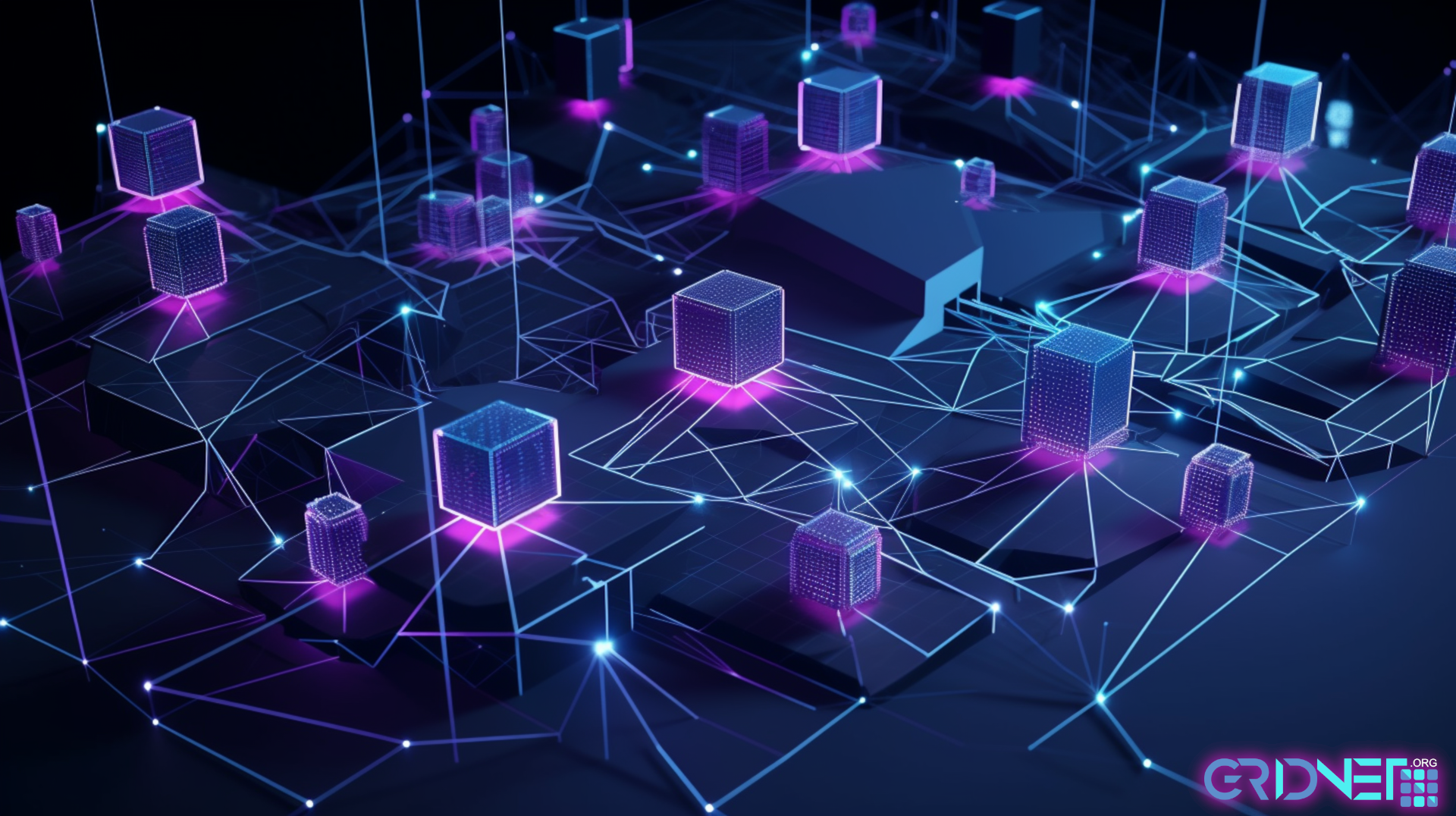
Once peer-to-peer networks learned to comprise banks.. then how to ‘think’.. they became so much more.
The poetry generating AI is open-source, contributed to by developers from various continents, and no single entity has full control over its training or operation. Now imagine the complexities of watermarking each AI-generated poem in this scenario, and tracking it back to the originating AI. It becomes a daunting, if not impossible task.
Who would be responsible for the watermarking? The developers who contributed to the AI, the server that hosts it (but wait a sec, there was no server to begin with..!) so would it be the Internet Service Provider, or Johnny who used the AI? And what if the poem, once published, is reshared, altered, or translated by other users?
This hypothetical scenario underscores the potential complexities that could arise with decentralized AI. And Decentralized AI – it is on its way.
The issues around watermarking, misidentification, and enforcement could become even more convoluted and challenging in a decentralized world. Thus, it becomes evident that we need novel and adaptive approaches to addressing AI regulation in an increasingly decentralized future.
Section 2: Decentralized AI: A New Path Forward
An introduction to the concept of decentralized AI.
Decentralized AI represents a paradigm shift in the architecture and operation of artificial intelligence systems. Traditionally, AI models are centralized, housed, and controlled by specific entities, typically tech companies. This centralization raises concerns about transparency, privacy, misuse, and single points of failure. Decentralized AI, on the other hand, seeks to address these challenges by distributing the computational tasks and decision-making authority across multiple nodes or entities. In a decentralized AI ecosystem, the training of machine learning models and the processing of AI tasks are carried out by a network of devices or nodes. This approach leverages the power of distributed computing and blockchain technologies. Each node in the network can contribute its computational resources, and in return, is rewarded, typically through a form of cryptocurrency or token. This incentivization structure encourages participation, thereby ensuring a robust and resilient network.
Decentralized AI allows for the democratization of AI development and use. It provides the opportunity for many individuals and entities to participate in and benefit from the AI economy. This not only helps to avoid the concentration of power but also facilitates the development of a more diverse range of AI models and applications.
Importantly, decentralized AI systems could potentially offer better privacy protections. Since the data and computations are distributed across the network, there is no central repository of information that can be hacked or misused. Furthermore, with appropriate use of encryption and secure multi-party computation techniques, individuals could contribute their data to AI models without giving up control over that data. In the context of generative AI and content regulation, a decentralized approach could open up new possibilities. Instead of relying on watermarks and detection mechanisms to track and control AI-generated content, a decentralized network could be designed to embed certain rules or principles into the AI generation process itself. This could provide a more robust and transparent way of ensuring responsible use of AI technologies, without hindering innovation.
Explanation of how decentralized AI overcomes the challenges of traditional watermarking regulation.
Getting back to the topic of watermarking, a decentralized AI system provides a novel perspective to tackle these problems. Instead of externally tagging content post-generation, it allows for an in-built system of content control and user responsibility.
- Proactive rather than reactive regulation: In a decentralized AI environment, the network’s nodes collectively manage the AI model’s training and output generation. This setup allows for proactive regulation, as the rules or principles governing content creation can be embedded in the training process itself. This mechanism contrasts with traditional watermarking, which is applied post-content generation and hence is fundamentally reactive.
- Reduced risk of misuse and abuse: The decentralization of AI models dilutes the concentration of power and reduces the risk of misuse. As the ownership and control of AI models are distributed across multiple nodes, the potential for abuse is significantly decreased.
- Enhanced transparency and accountability: In a decentralized AI system, all nodes share responsibility for the AI’s outputs. Blockchain technologies ensure that every contribution to the AI’s training and every modification to its underlying architecture is recorded, creating an auditable trail. This increased transparency could enhance accountability and trust within the system.
- Adaptability and resilience: Decentralized AI systems can quickly adapt to new situations or challenges. For example, if a particular type of AI-generated content becomes problematic, the network can collectively decide to adjust the AI model’s parameters or training data to address this issue. This dynamic adaptability contrasts with traditional watermarking systems, which may struggle to keep up with the rapid pace of AI advancements.
- Better reinforcement towards user expectations: As discussed in the context of the GRIDNET OS, a decentralized AI system can enable a more tailored user experience. By creating sub-grids or realms, each with its distinct features and reinforcement learning parameters, the system can better adapt to specific users’ expectations.
Without a doubt, by embracing a decentralized approach, we could move towards a more effective, proactive, and user-centric model for managing AI-generated content. While this doesn’t mean that all challenges would disappear, decentralization of AI certainly opens up additional compelling avenues to peruse, instead of traditional watermarking regulations. As already discussed, watermarking, as a method to regulate AI-generated content, presents inherent challenges, particularly concerning text-based content.
Section 3: Unleashing the Potential of GRIDNET OS
The features and capabilities of GRIDNET OS can help significantly in the journey towards achieving truly decentralized AI, thereby addressing and overcoming many of the issues and challenges associated with watermarking. Here’s how:
- Decentralized User-Interface Mechanics and Computing: These two features allow developers and users to interact with AI applications in a decentralized manner. AI models can be trained and operated across a network of nodes, eliminating the need for central points of control, interactively. This decentralization can reduce the risk of AI misuse as each node in the network can verify the operations and outputs of an AI model, helping to ensure transparency and authenticity.
- Decentralized Storage and File-System: The decentralized storage and file-system of GRIDNET OS provide a robust and secure way to store AI-generated content. This decentralized storage system makes tampering with AI-generated content more challenging, and increases the difficulty of generating and spreading deepfakes, thereby addressing some of the key concerns that watermarking aims to solve.
- Efficient Off-The-Chain Transactions: Efficient off-the-chain transactions can expedite the detection and flagging of potentially harmful or misleading AI-generated content. This speedy response can prove invaluable in managing and minimizing the spread of such content, offering a proactive approach rather than relying solely on watermark identification.
- Built-In Decentralized Voting Constructs: The decentralized voting constructs built into GRIDNET OS can provide a democratic method for managing content in the network. If there’s a dispute about the authenticity or appropriateness of certain AI-generated content, a vote could be held to decide the best course of action.
- Decentralized Identity with Stake Concept: The ‘Stake’ concept tied to decentralized identity could incentivize responsible behavior in the network. If an individual or entity tries to spread misleading AI-generated content or misuses the network, they risk losing their stake.
Through these innovative features and capabilities, GRIDNET OS not only addresses the inherent issues of watermarking AI-generated content but also offers a proactive, decentralized, and democratized approach to regulating AI. By making the network participants collectively responsible for AI behavior, it creates a self-governing system where misuse can be readily identified, flagged, and dealt with. This aligns with the ultimate goal of fostering transparency, trust, and fairness in the AI landscape.
Section 4: Decentralized Stable Diffusion in AI Generative Art
Explanation of the decentralized stable diffusion process.
The decentralized stable diffusion process would form an essential aspect of the proposed alternative to watermarking. As part of a decentralized AI algorithm system, it would play a key role in ensuring the integrity, security, and robustness of AI models within a decentralized network. In traditional centralized AI systems, a single authority or entity controls the development and updates of an AI model, leading to a potential single point of failure or misuse. However, in a decentralized setup, the stable diffusion process allows for distributing the AI model’s updates and improvements across the network, fostering a more resilient and secure ecosystem.
- Robustness and resilience: The decentralized stable diffusion process would enhance the system’s robustness by distributing the AI model across the network. Each node holds a version of the AI model and contributes to its learning and improvement. This setup prevents single points of failure, ensuring that the system remains functional even if individual nodes fail.
- Collective Learning and Improvement: In the stable diffusion process, all nodes participate in training the AI model and share the improvements with the rest of the network. This collective learning approach allows the AI model to learn from a diverse set of data and experiences, leading to more robust and generalizable models.
- Security and Privacy: The decentralized nature of the decentralized stable diffusion process also would improve the security and privacy of the system. Since each node only holds a portion of the data, the risk of data breaches or misuse is significantly reduced. Furthermore, each update or modification to the AI model is recorded on the blockchain, ensuring transparency and accountability.
- Flexibility and Scalability: The decentralized stable diffusion process would be highly scalable and flexible. It could easily accommodate new nodes or data sources, allowing the AI model to grow and evolve over time.
- Improved Decision-Making: Lastly, the stable diffusion process can support decentralized decision-making. By using built-in voting constructs within autonomous enforcement of poll results, the network can collectively decide on significant updates or modifications to the AI model.
The decentralized stable diffusion process could facilitate a more secure, robust, and democratic approach to developing and improving AI models. By incorporating this process, we could mitigate the challenges posed by traditional watermarking regulations, leading to a more balanced and resilient AI ecosystem.
Discussion on how this process can be beneficial in a decentralized AI environment.
Decentralized AI offers a fresh perspective on how we design and interact with artificial intelligence systems, and it brings several key advantages that help overcome the shortcomings of the traditional centralized approach. Let’s discuss how decentralization can transform the AI landscape.
- Innovation and Diversity: In a decentralized AI environment, the algorithms used for AI development are open and accessible. This encourages a vast network of developers to contribute their unique ideas and improvements, leading to a more innovative and diverse AI ecosystem. The process fosters the creation of specialized AI models that cater to a wide variety of applications and user needs.
- Transparency and Trust: Unlike black-box models where decision-making processes are hidden, decentralized AI promotes transparency. As algorithms and data are openly accessible, it becomes easier to audit AI systems, understand their decisions, and identify any biases. This transparency builds trust among users, stakeholders, and regulatory bodies.
- Resilience and Security: Decentralized AI systems are inherently robust and resistant to single points of failure. The distributed nature of the system provides a robust defense against hacking or data tampering. Furthermore, each user in the network retains control over their data, reducing the risks associated with large centralized data repositories.
- Crowd-Sourced Learning and Improvement: In a decentralized AI environment, machine learning can be a collective effort. User interactions across the network contribute to the continuous learning and improvement of AI models. This feedback loop can enhance the accuracy and relevance of AI responses, leading to better user satisfaction.
- Governance and Ethics: Decentralized AI systems could implement decentralized voting mechanisms to determine network rules, improving governance and ethical oversight. For example, users could vote to ban specific types of content or behaviors, ensuring the system aligns with societal norms and values.
- Economic Incentives: Decentralized AI can also foster new economic models. For instance, users contributing their data or computational resources for training AI models can be rewarded with cryptocurrencies or tokens. This not only incentivizes participation but also paves the way for a more equitable distribution of AI-generated wealth.
In a world where AI is becoming increasingly influential in our daily lives, a decentralized approach provides a pathway that is transparent, inclusive, and innovative. This process is not only beneficial but also necessary for creating AI systems that truly serve the diverse needs of humanity.
Section 5: Towards a More Democratic and Efficient AI Ecosystem
As we venture into the realms of decentralization and the complex nuances of artificial general intelligence (AGI) systems, crucial decisions need to be made regarding the network architecture, data storage and sourcing for training. Transformer architectures have proven to be highly parallelizable, a fact that propelled models like GPT to prominence. Yet, in a decentralized setting, traditional distribution systems like BOINC, where a central server sends job packets to distributed clients, may not offer the perfect solution. Ideally, we aim to establish a more equitable network, akin to blockchain systems, where all nodes share equal status.
Indeed, in this new order, we might need to establish a concept akin to ‘proof of training,’ where a language model node indicates that a specific text has been trained locally and is ready to disseminate the updated weights to other nodes. Here, standard datasets such as The Pile could serve as training material.
When it comes to the hardware question, a widespread lack of GPUs can pose a problem. However, there is a growing dialogue around using alternative hardware for training large language models. For example, companies like Cerebras have received attention for their efforts in this area. This discussion brings us to the matter of incentives. Once an AGI model has been trained, it essentially belongs to the entity that accomplished the task. However, not everyone can host a model due to hardware limitations. For non-hosting node-providers, one can envision a system where they validate training chunks and are given a limited number of queries each day as a form of compensation.
This takes us into the three core processes behind interacting with an AI like ChatGPT: training, fine-tuning, and inference. The first, training, is an intensive step involving substantial data loading. Fine-tuning lends the model its distinct character, and inference allows the actual generation of text, such as in a chat or producing a piece of fiction. But what if we could optimize these processes further? We’ve seen the rise of initiatives working towards running large models locally, often without networked parallelism, and focusing on local inference and some fine-tuning. As corporations are building projects with up to 300 billion parameters, the potential of utilizing decentralized AI grows.
In this context, GRIDNET’s off-the-chain incentivization framework could offer a potential solution for incentivizing decentralized GPU computing necessary for training decentralized AI algorithms. However, we must emphasize that this is not about creating a marketplace for AI but rather ensuring that we collectively harness the potential of AI by decentralizing its training and operation.
To meet the computational demands and ensure consistent performance, modest but critical goals have been set for this endeavor, including maintaining high system uptime and creating a fully open-source environment. Cost considerations must also be acknowledged, but with a sufficient user base, these expenses become manageable. As we move forward, we must remember that we are not just building technology; we are shaping the future of AI. Decentralized AI holds great promise, and with the right incentives and infrastructure in place, we can ensure its potential is fully realized for the benefit of all.
Discussion on the benefits of a decentralized approach to AI, emphasizing freedom from restrictive regulation and the potential for AI innovation.
The shift to a decentralized approach for AI development and deployment brings a host of benefits, with an emphasis on enabling more freedom for innovation and participation. As we traverse an era where AI technology’s impact on our society and personal lives continues to grow, the idea of a more democratic and open AI development ecosystem becomes increasingly attractive.
- Freedom to Innovate: Decentralized AI facilitates a vast platform for innovation. Instead of a few centralized entities controlling the advancement of AI technology, a decentralized setup allows anyone in the network to contribute to AI models’ growth. This freedom sparks creativity, leading to diverse AI applications and solutions.
- Freedom from Monopolies: In a decentralized AI network, no single entity can monopolize the AI technology. This freedom prevents the concentration of power and its potential misuse, fostering a more equitable AI environment.
- Freedom of Access: With decentralized AI, there is a democratization of access to advanced AI technology. Irrespective of geographical location or economic status, any individual or entity in the network can access and use the AI model.
- Freedom to Participate: In a decentralized AI environment, every participant in the network has the opportunity to contribute to the AI model’s development. This contribution can range from providing training data to optimizing the AI model’s architecture, ensuring a more inclusive AI development process.
- Freedom to Control Data: Perhaps the most crucial aspect is the freedom to control one’s data. In a decentralized setup, each participant maintains the ownership of their data, which contrasts with centralized AI systems where data privacy can often become a significant concern.
By integrating decentralized AI into the GRIDNET OS, we leverage the inherent benefits of decentralized systems, including the decentralized stable diffusion process, which will create a more robust, transparent, and inclusive AI development environment. Consequently, the proposed system significantly overcomes the challenges presented by traditional AI watermarking, aligning with the rapid growth and expansion of AI technology without compromising individual freedom and innovation.
A case for decentralized voting constructs in maintaining the well-being of the network.
In the realm of decentralized AI, the application of decentralized voting constructs becomes vital in upholding the integrity and fair functioning of the network. By integrating a voting mechanism into the system, we can foster a democratic environment that promotes collective decision-making, effectively circumventing potential disputes, and ensuring the network’s well-being.
- Democratic Decision-Making: Decentralized voting constructs allow for democratic decision-making in the network. Each participant has the right to vote on important matters related to the AI model’s development, including changes to the model’s architecture, introduction of new features, and data usage policies. This democratic approach ensures that no single entity can dictate the course of AI development.
- Resolving Disputes: Disagreements and disputes are inevitable in any community. Decentralized voting constructs provide an unbiased and democratic mechanism to resolve such issues. Through voting, the network’s participants can collectively decide on the best course of action, ensuring fairness and maintaining harmony within the network.
- Protecting the Network: Decentralized voting constructs also act as a protective measure for the network. If any harmful or unethical practices emerge, the network’s participants can collectively decide to halt or rectify such actions through voting. This process safeguards the network from potential misuse of AI technology.
- Encouraging Participation: The availability of a voting mechanism encourages active participation from the network’s members. Knowing that their opinion holds value and can impact the AI model’s development motivates individuals to contribute more actively to the network.
- Autonomous Enforcement: The results of these polls are autonomously enforced within the network. This feature ensures that the collective decision is implemented effectively, adding another layer of trust and transparency in the system.
Incorporating such decentralized voting constructs within the GRIDNET OS would enable a truly decentralized and democratic AI development environment, taking us a step closer to a more equitable and inclusive AI future. The benefits of such an approach go beyond the AI development process, affecting societal aspects like governance and decision-making at a broader scale.
Quantum Possibilities: Towards an Unfathomable Future
The landscape of artificial intelligence and computing is being reshaped continuously and at an accelerating pace. Today, as we stand at the brink of another technological revolution, we find ourselves contemplating possibilities that we wouldn’t have believed mere months ago. Quantum computing, an area once confined to theory and laboratory experimentation, is rapidly emerging as a potent tool that can redefine the horizons of artificial intelligence. Recently, a Chinese team of scientists announced a staggering breakthrough. Their quantum computer, aptly named Jiuzhang, after a 2,000-year-old Chinese math text, demonstrated performance capabilities that surpass our wildest expectations. This quantum computing device, the team reported, could perform certain tasks commonly used in artificial intelligence a staggering 180 million times faster than the world’s most powerful classical supercomputer.
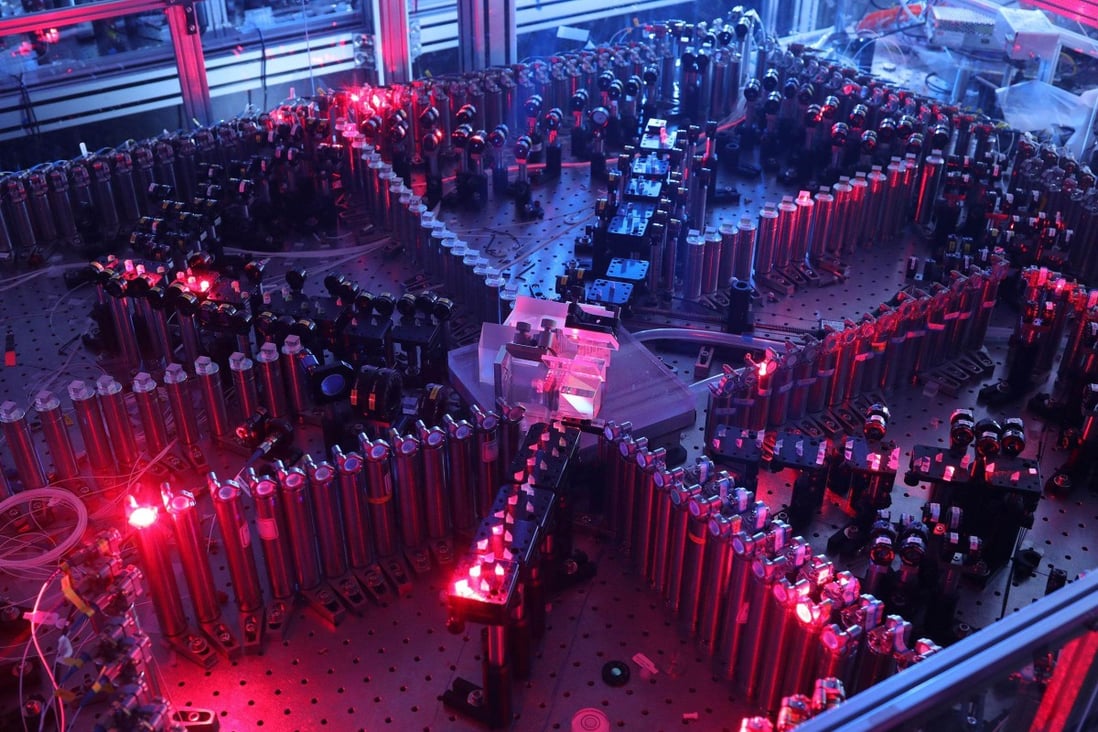
In research using China’s Jiuzhang computer, scientists implemented and accelerated two algorithms – random search and simulated annealing – that are commonly used in the field of AI. Photo: University of Science and Technology of China
The experiment involved the quantum computer solving a problem that poses significant challenges to classical computers. Using over 200,000 samples, Jiuzhang took less than a second to accomplish a task that would have taken nearly five years on the world’s fastest classical supercomputer. The implications of this leap in computational power are immense, with potential applications ranging from data mining to biological information processing, network analysis, and chemical modeling research.
These revelations invite us to contemplate a future where artificial general intelligence (AGI) is powered by quantum computing. It also nudges us towards understanding that the AI of the future might be more diverse and versatile than we currently imagine. Furthermore, such advancements might soon bring us to the point where we employ quantum-assisted computers to solve complex problems, potentially prolonging our lifespan, an idea that, for some, may stir up religious or ethical concerns.
While quantum computing is still in its nascent stages and comes with its share of challenges, it undeniably opens up vistas of opportunities. Quantum computers, unlike their classical counterparts, can process multiple states simultaneously, potentially making them faster and more powerful. However, this quantum advantage comes with a trade-off – the technology is fragile and can be easily disrupted. Jiuzhang, however, deviates from this narrative. It employs light as the physical medium for calculation, offering enhanced stability and doesn’t require extremely cold environments to function effectively.
This quantum leap in computing serves as a stark reminder of the importance of open-mindedness in technological innovation. As we advance towards a future replete with decentralized AI, we must be mindful of the potential role of quantum computing. We must refrain from being hemmed in by rigid regulations that may stifle progress. It’s crucial to strike a balance between ethical considerations and technological advancement, ensuring we maximize the potential of AI without compromising on the values we hold dear. This balance would not only pave the way for harnessing the power of quantum computing for the betterment of humankind but would also ensure that the evolution of AI stays on a path that is beneficial for all.
Embracing Quantum-Enhanced AI: A Paradigm Shift in the Making
Quantum computing is poised to transform AI as we know it, powering the next wave of profound innovations and scientific breakthroughs. With the advent of machines like Jiuzhang, we find ourselves staring into a future of AI that is not merely an improvement on the present but a revolutionary leap forward. This is a leap that has the potential to redefine our very understanding of the universe and our place within it. There are valid concerns about the introduction of quantum computing and its interplay with artificial intelligence. Quantum computers, with their seemingly boundless power, could exacerbate the already complex issues surrounding AI, such as privacy, data security, and potential misuse. While these concerns must not be overlooked, they also should not stifle the growth and development of this game-changing technology. A measured and thoughtful approach to policy and regulation is needed to ensure that the advent of quantum-enhanced AI is met with readiness and not resistance.
One area that is ripe for disruption by quantum computing is healthcare. By harnessing the power of quantum-enhanced AI, we could revolutionize medical research, diagnostics, and treatment. This could lead to more accurate diagnoses, personalized treatment plans, and even the potential to prolong human lifespan. While the ethical and religious debates around such prospects are inevitable, it’s important to consider the larger picture – the potential for enormous societal benefits. The advent of quantum-enhanced AI could also significantly impact the financial sector, logistics, cybersecurity, and even climate modeling, facilitating solutions to some of the most pressing global challenges. However, to realize this future, we must navigate the delicate balance between innovation and regulation. As we stride into this exciting yet uncharted future, it is essential to consider the decentralized AI approach, with platforms like GRIDNET OS leading the charge. The emphasis on decentralization and open-source methodologies could prove crucial in harnessing the potential of quantum-enhanced AI while ensuring transparency, collaboration, and broad access.
In this vein, it’s worth revisiting the words of physicist Niels Bohr: “Those who are not shocked when they first come across quantum theory cannot possibly have understood it.” Quantum computing, with its power to disrupt and transform, promises a future that may shock, surprise, and eventually, transcend our current understanding of what’s possible. As we stand on the precipice of this exciting new era, let us embrace the shock, navigate the challenges, and journey into a future shaped by the untapped power of quantum-enhanced AI.
Bracing for the Quantum Leap: An Open-Source Future
The open-source movement, characterized by collaborative development and unrestricted access, serves as a possible model for the ethical and effective harnessing of quantum-enhanced AI. As quantum computing intersects with AI, new horizons open up, ushering in unparalleled possibilities. But with this unfathomable power comes the need for robust safeguards, shared responsibility, and a commitment to collective benefit. In an open-source environment, transparency and accountability become ingrained in the development process. Coupled with the distributed nature of blockchain systems like GRIDNET OS, we stand a better chance of avoiding the pitfalls of centralized control, misuse, and single-point failures.
Take, for instance, the healthcare industry. Open-source, quantum-enhanced AI could expedite drug discovery, genetic research, and patient care, transforming it from a reactive to a proactive practice. The idea of quantum computers aiding in prolonging human lifespan, though seemingly radical, is a distinct possibility within our grasp. Religious, ethical, and societal debates will inevitably surround these advancements. Yet, we must remember that progress is born of open-mindedness and courage. Advancements that were once unimaginable – like using AI algorithms to simulate protein folding or leveraging quantum physics to build superfast computers – are today’s reality. Therefore, to shun the potential benefits of quantum-enhanced AI due to fear of change or strict regulations would be a disservice to human advancement.
Quantum-enhanced AI brings us to the brink of a future that we cannot yet fully fathom. While the challenges are significant, the potential rewards could redefine our world in unimaginable ways. To navigate this future, we must remain agile, maintaining a balance between innovation and regulation. We must foster an environment where developers, researchers, and users co-exist and collaborate, exchanging ideas and challenging boundaries.
Let us not be hamstrung by fear, but rather let us foster a spirit of exploration and innovation. After all, our future might just be a quantum leap away.
The Paradox of Regulation: AI Watermarking and the Inevitable Future
While it is understandable that governments strive to protect their citizens through regulations, the dynamics of technology development often outpaces their efforts. This seems to be the case with the attempt to enforce AI watermarking. It’s not merely an issue of feasibility; it’s a clash with the very nature of open algorithms and computational engines. The challenges of such an endeavor could be equated to trying to tame the Internet in its early days – a daunting, if not impossible, task.
The attempt to watermark AI-generated content can be seen as a somewhat desperate and futile effort to halt the inevitable advancement and proliferation of artificial intelligence. It’s an attempt to impose restrictions on something inherently unrestricted – like trying to contain water with a sieve. The negative impacts of this approach are not to be overlooked.
Consider OpenAI’s ChatGPT, one of the groundbreaking AI technologies of our time. If it were to be subjected to such constraints, it would not only hurt the company behind it but also negatively impact the wider ecosystem of AI innovation. Rather than fostering progress, such measures could stifle creativity, hinder research, and redirect resources away from productive pursuits. The monetary flows that would have been invested in further AI development might instead be redirected to jurisdictions without such legal constraints, diluting the intended effect of the regulations. However, let us look beyond the present and cast our gaze toward the horizon. The advances in quantum computing are moving at a rapid pace. The recently developed Chinese quantum computer, Jiuzhang, performs tasks commonly used in artificial intelligence 180 million times faster than the world’s most powerful classical supercomputer. The implications of this breakthrough are profound and far-reaching.
Even without the advent of quantum computers, the rise of decentralized AI networks seems inevitable. The push towards decentralization is gaining momentum, spurred by the inherent advantages it offers in terms of flexibility, resilience, and independence from central points of failure or control.
The future is ripe with possibilities, and it seems increasingly clear that decentralized AI networks, potentially powered by quantum computing, are a part of that future. Rather than trying to impede this progress, it would be more constructive to find ways to guide it responsibly, to ensure that these powerful technologies are used for the benefit of all. The principle of decentralized voting can play a key role here, offering a mechanism to navigate the collective decision-making processes needed to shape the future of AI.
In this context, the GRIDNET Decentralized Operating System (GRIDNET OS) comes to the fore. With its off-chain incentivization framework, it could be an excellent candidate to host the decentralized GPU computing needed for these AI algorithms. The platform is designed to ensure openness, freedom, and fairness – principles that will be crucial in shaping a positive AI-powered future. The message is clear: rather than attempting to halt the tide of progress, we should focus on guiding it. Rather than trying to watermark AI content, we should aim to create a regulatory environment that supports the responsible development and use of AI. This future-oriented perspective is not just an option; it’s a necessity if we want to harness the full potential of AI and quantum computing in the service of humanity.
The Conundrum of Control: Understanding the Regulatory Perspective
The stance of a government seeking to limit or control AI, while seemingly oppositional to the spirit of innovation, is not without a certain rationale. Governments are tasked with maintaining order, ensuring safety, and safeguarding the public interest. They deal with a complex array of considerations, ranging from national security to economic stability, from public welfare to ethical concerns. Thus, their instinct to regulate, to control, to set boundaries is not merely an expression of power but often stems from their responsibility to the public.
When it comes to AI, governments are grappling with a double-edged sword. On one hand, AI presents enormous potential for societal advancement. It promises to revolutionize industries, improve efficiencies, and unlock new solutions to age-old problems. On the other hand, AI also poses substantial risks, such as privacy violations, algorithmic bias, malicious use, job displacement, and even threats to national security. Striking the right balance between harnessing the benefits and mitigating the risks is a complex and delicate task. From this viewpoint, the desire to enforce AI watermarking or any form of AI regulation is an attempt to exercise control over an emerging technology that is both promising and perilous. It represents a government’s effort to keep pace with technological advancement, to adapt its regulatory frameworks to a rapidly evolving landscape.
Technically, enforcing such control would necessitate a departure from the openness of algorithms that currently characterizes the AI field. It would mean transitioning to closed-source algorithms, which could be subject to licensing and regulation. This could indeed be a plausible scenario for entities like OpenAI, which currently owns a highly trained and effective artificial brain. The implications of moving toward closed-source algorithms are far-reaching. This approach could stifle innovation, limit the democratization of AI, and even create a technological monopoly. Moreover, it fails to consider the trend toward decentralization, which inherently resists such control.
As AI becomes increasingly decentralized, the effectiveness of regulatory measures such as AI watermarking diminishes. A decentralized network, once properly implemented, could potentially exceed the capabilities of any single entity’s AI, including that of OpenAI. These networks, free from the limitations of centralized control, can learn, grow, and innovate in ways that a closed-source, tightly controlled AI cannot. In light of these considerations, the regulatory perspective faces a significant challenge. How can governments protect the public interest without stifling innovation and limiting the potential of AI? The solution likely lies in a balance between regulation and freedom, between control and openness. But finding this balance in the dynamic, complex, and largely uncharted territory of AI will be a formidable task.
Harari’s Vision vs. Today’s Reality: The Rapid Ascendancy of AI
When Yuval Noah Harari laid out his vision of the future in his acclaimed books, “Sapiens,” “Homo Deus,” and “21 Lessons for the 21st Century,” he charted a provocative path. Harari portrayed a world where AI and biotechnology would transform societies, economies, and even what it means to be human. The Israeli historian posited that these technologies could end up creating new classes of individuals, governed by data and algorithms.
While his predictions were met with a mix of fascination and skepticism, it is striking to note how rapidly the reality is catching up with, and even surpassing, Harari’s vision. Even Harari, with his futurist’s gaze, might not have foreseen the speed and scale at which AI, in the form of tools like ChatGPT and Midjourney, would pervade our lives. These technologies have transformed the landscape of communication, content creation, and information processing, altering how we interact with digital spaces and each other.
The current state of AI indeed highlights how Harari’s provocative ideas are unfolding in real time, but with a new layer of complexity – the emergence of decentralized networks. Harari’s discourse revolved primarily around the impact of centralized entities wielding the power of AI and biotechnology, overlooking the potential of decentralized systems. As government bodies and centralized entities grapple with the idea of regulating AI technologies, an alternative path is emerging through decentralized networks like GRIDNET OS. These networks offer a compelling model that combines the openness and innovation of AI with a structure that resists monopolistic control.
GRIDNET OS presents itself as a safe haven for innovation and access, fostering an environment where AI technologies can grow and evolve without being shackled by centralized controls or restrictive regulations. It embodies a model of technological evolution that not only aligns with the spirit of innovation championed in Harari’s books but also goes a step further, adding a new dimension of decentralization to the discourse.
In this rapidly transforming landscape, the likes of GRIDNET OS serve as a reminder that while the future might hold uncertainties, it also holds the potential for unprecedented innovation and democratization. As Harari’s vision unfolds, it is these decentralized technologies that are pushing the boundaries of what we thought possible, ushering us into a future where AI is not only powerful but also open, accessible, and shared among all.
Biotechnology and Life Extension: The Controversial Yet Promising Role of Decentralized AI
As we tread further into the twenty-first century, we stand on the brink of tremendous breakthroughs in biotechnology and life extension – fields that have sparked intense debate due to their profound ethical and societal implications. Within this discourse, a particularly intriguing proposition emerges: the application of decentralized AI.
Decentralized AI, with its inherent traits of open access, robustness, and collective learning, can play a transformative role in these domains. A compelling use case lies in molecular analysis over time, a crucial aspect of understanding and potentially extending the human lifespan.
Consider the complexity of biological systems: Our bodies house a staggering number of molecules, each interacting, changing, and influencing our health over time. Detecting and understanding these changes is a monumental task, one that far exceeds human capacity. Traditional centralized AI systems have been employed to tackle this complexity, but they face limitations in data access, adaptability, and scalability. Here’s where decentralized AI could make a difference. By employing a distributed network of AI systems, each capable of learning and adapting, we can simultaneously analyze a vast number of molecular changes. This decentralized approach not only scales up the analysis but also ensures a diversity of data and perspectives, enhancing the robustness and reliability of the findings.
The relationship detection capability of AI can uncover patterns and connections that might be invisible to human researchers, opening up new avenues in our understanding of molecular biology and aging. Moreover, these insights could inform interventions, from lifestyle changes to personalized medicines, that could extend healthy human life. However this application of AI is not without controversy. The notion of life extension itself raises profound questions about our societal structures, ethics, and the very nature of human existence. Furthermore, the usage of personal biological data in decentralized AI networks will necessitate robust protocols to ensure privacy and data security.
Yet, despite these challenges, the potential of decentralized AI in biotechnology and life extension is undeniable. By enabling us to grapple with the complexities of biological systems, it could bring us a step closer to the once-unthinkable goal of extending human life. As we navigate these uncharted territories, the key will lie in ensuring that these technologies are developed and deployed responsibly, with a firm commitment to ethical principles and the collective good.
A Scientific Proposition: Decentralized AI as a Key to Life Extension and Aging Reversal
In the realm of molecular biology and life extension, the integration of decentralized AI could revolutionize our approach to unraveling the mysteries of aging. The mechanisms of aging are multi-layered and intertwined, requiring comprehensive and adaptive analytics that the distributed network of AI systems can offer. Here, we propose a methodology that leverages decentralized AI to uncover the keys to life extension and aging reversal.
Step 1: Data Collection and Preparation
The decentralized network collects molecular data from a multitude of sources, including genomic sequencing, metabolomics, proteomics, and more. AI nodes in the network might specialize in specific types of data, ensuring an expert-level analysis of diverse biological information. Personal biological data, if shared voluntarily and ethically, could offer invaluable insights into individual variations in aging.
Step 2: Distributed Analysis
Each AI node in the decentralized network is responsible for analyzing a portion of the dataset. By distributing the task, the network can manage the vast volumes of molecular data, significantly reducing the time required for analysis. Moreover, the network can collectively adapt and learn, refining its algorithms as it processes new data.
Step 3: Pattern Recognition and Hypothesis Generation
Through machine learning algorithms and AI capabilities, the network identifies patterns and relationships within the molecular data. This process could reveal correlations between specific molecular changes and aging, providing clues to potential targets for life extension interventions. The network can generate hypotheses based on these findings, proposing avenues for further exploration.
Step 4: Hypothesis Testing and Validation
The proposed hypotheses are tested in computational models or laboratory settings. AI nodes specializing in modeling and simulation can predict the potential impacts of modifying identified molecular targets. These predictions are then validated through experimental testing. It’s worth noting that AI doesn’t replace human scientists in this step but significantly augments their capabilities by enabling high-throughput, precise, and complex experiments.
Step 5: Knowledge Integration and Dissemination
The findings, whether they support or contradict the initial hypotheses, are integrated into the network’s collective knowledge. This continual learning process refines the AI algorithms, enhancing their predictive accuracy over time. The results are disseminated throughout the network, allowing other AI nodes to benefit from the findings.
Step 6: Intervention Design and Personalization
Based on the validated findings, the AI network can suggest potential interventions for life extension or aging reversal. These might include pharmacological targets, genetic modifications, or lifestyle changes. Furthermore, AI can personalize these recommendations, taking into account individual molecular profiles and health status.
The potential of decentralized AI in unlocking the secrets of aging and life extension is immense. However, the implementation of this methodology must be accompanied by rigorous ethical standards, robust data security, and a commitment to scientific integrity. As we explore this pioneering approach, we are not merely harnessing the power of AI but stepping into a new era of understanding and potentially influencing the biology of aging.
Navigating the Future: Government, Religion, and Technological Revolution
The technological advancements enabled by decentralized AI, especially in domains such as biotechnology and life extension, will inevitably cause ripples in societal structures, particularly in government and religious institutions. These entities, grounded in long-established norms and values, often find it challenging to reconcile their perspectives with the rapid pace of scientific and technological innovation.
From the government’s standpoint, the use of AI in biotechnology can introduce a plethora of complex issues related to ethics, privacy, and security. Regulators will have to grapple with creating guidelines that protect individual rights and societal interests without stifling the progress of this potentially transformative field. There could be widespread debate over the extent of control that individuals should have over their genetic information and the modifications they might choose to make. The implications for health insurance, medical practice, and even societal structures could be profound. Religious institutions may face even deeper existential questions. Many religious traditions have long-held beliefs about the sanctity of life and the natural order of the world. The notion of human intervention in the process of aging and life extension could challenge these beliefs, leading to intense debates and potential resistance.
It is essential to remember that humanity has navigated similar crossroads in the past. Technologies such as electricity, space travel, or medical imaging, which were once unimaginable or even considered heretical, are now integral parts of our lives. This evolution didn’t occur without resistance, but our collective commitment to progress and human well-being ultimately prevailed.
As we stand on the precipice of a new era, we must ensure that our drive for progress is not hindered by outdated perspectives or fear of the unknown. The same way few today care that ancient deities like Zeus or Ra were once believed to govern natural phenomena, we should not let historical or cultural beliefs limit our pursuit of knowledge and improvement. We are humans – we have the capacity to adapt, to learn, and to create a better future for ourselves. Indeed, we do not wish to “go to heaven too early” due to misguided or uncontrolled genetic modifications. Instead, we aim for a careful, conscious manipulation of our biology that may enhance our quality of life and extend our time on this planet. We are not merely fighting against aging or disease; we are fighting for our right to live fully and meaningfully. Certainly, the journey towards the future will be complex and challenging. It will require an open dialogue among scientists, ethicists, policymakers, religious leaders, and the public. As we navigate this path, our guiding principle should be our shared commitment to human dignity, well-being, and the unceasing exploration of our potential.
But the question is – are Watermarks of any kind to stop AI aided research on long-living? Are Watermarks to make algorithms secret ?
Conclusions
Summary of the issues with the current AI regulations and the potential of decentralized AI to overcome these issues.
Current AI regulations, specifically the implementation of watermarking for text-based generative AI, present a host of challenges that have sparked concern and debate among policymakers and stakeholders worldwide. While watermarking may seem like a practical method to identify and control the spread of AI-generated content, its application to text-based content is fraught with complexities and potential misuses. Existing watermarking methods for text-based AI content are imperfect, with high chances of false positives and negatives. The inherent difficulty of embedding watermarks in text without altering its meaning gives rise to these issues. Furthermore, the ease of manipulating such watermarks exacerbates the problem, making them an unreliable mechanism for control.
The challenge extends to the enforceability of watermarking regulations. Considering the potential for real harm when human-generated content is falsely flagged as AI-generated, implementing such regulations can lead to unjust consequences. In addition, the possibility of adversaries intentionally mimicking watermarks to mislead or cause harm adds another layer of complexity. The decentralized AI approach, embodied by the proposed GRIDNET OS, presents a promising solution to these challenges. By utilizing decentralized open AI algorithms, including the novel concept of decentralized stable diffusion, we can move towards a system that prioritizes transparency and accountability without impinging on the freedom of expression. Decentralized AI allows for a democratic, participatory environment where collective decision-making is possible through voting constructs. This setup helps maintain the well-being of the network, resolving disputes, and ensuring fair and ethical practices.
The decentralized stable diffusion process would mitigate the issues related to watermark manipulation and misuse. By decentralizing the AI generation process, the risk of a single entity exerting undue influence or causing widespread harm is substantially reduced. While the current AI regulations pose significant challenges, the potential of decentralized AI, as exemplified by the GRIDNET OS, offers an alternative path forward. This approach, centered on transparency, democratic decision-making, and collective ownership, could reshape the future of AI development and its integration into society.
Call to action for embracing the proposed decentralized approach to ensure unhindered progress in AI.
Given the complexities and challenges associated with current AI regulations, it is crucial that we explore and embrace alternative pathways that promise a more equitable and transparent future for AI. One such promising avenue is decentralized AI, which offers substantial benefits in terms of transparency, accountability, and shared governance. As we delve deeper into the era of artificial intelligence and machine learning, the importance of a decentralized approach becomes even more paramount. The capacity to foster innovation while maintaining ethical standards and minimizing potential misuse of AI technology is an opportunity we cannot afford to miss.
With quantum-enhanced AI opening new vistas, it’s crucial that we chart this largely unexplored territory with due care and responsibility. Given the enormity of the potential and the risks involved, the governance of this emergent technology should not rest solely in the hands of a few entities or organizations.This is where the potential of decentralized networks like GRIDNET OS comes to the fore. Through such platforms, we can strive for a distributed governance model, one that allows for the collective wisdom of diverse stakeholders to guide the course of this technology. This decentralization, inherently democratic and inclusive, can ensure a fair and balanced approach to quantum-AI development and use. In the face of quantum advancements, regulations must be forward-thinking, evolving in tandem with technology. They should not stifle innovation but instead foster a conducive environment for growth and exploration. They must also be flexible, allowing for course corrections based on new insights, experiences, and societal needs.
Decentralized networks can also serve as effective platforms for collective decision-making. Using mechanisms like consensus algorithms, stakeholders can participate in deciding the rules of the game, contributing to the design of regulations, and monitoring compliance. Such participatory governance can help build trust, foster a sense of ownership, and ensure that the benefits of quantum-enhanced AI are widely distributed. In this quantum-infused future, the role of the developer community is more critical than ever. You, the architects of this new world, have the opportunity and responsibility to shape it. Your code is not just a set of instructions for machines; it’s the blueprint for the society of tomorrow.
The quantum leap is within sight, yet we’re still on the cusp of fully understanding its implications. As we tread these uncharted waters, let’s hold steadfast to the principles of openness, collective wisdom, and shared benefit. The journey might be fraught with uncertainties and challenges, but if navigated with care, the rewards could be as monumental as the quantum leap itself. So, let’s embrace this journey, one qubit at a time.
To actualize this potential, we call on all stakeholders in the AI and machine learning ecosystem, particularly developers and researchers, to consider the opportunities presented by the decentralized approach, embodied in this case by the GRIDNET OS. This is not just about creating sophisticated AI models; it is also about shaping an environment where AI can flourish in a responsible and controlled manner. Developers across the globe are invited to contribute to the development and implementation of the GRIDNET OS. Your expertise, creativity, and passion can help propel the vision of a decentralized AI future into reality. Let’s work together to build an AI ecosystem that is as diverse, inclusive, and ethical as the world we aspire to create.
Join us in this transformative journey. Together, we can shape the future of AI, ensuring its progress is not hindered but rather empowered by our collective efforts and the principles of decentralization.
Evolution Always Finds a Way
As we move forward in our quest to understand and shape artificial intelligence, it’s important to remember that evolution is a persistent force, always finding a way to adapt and thrive amidst change. In the realm of AI, this evolutionary process calls for our collective creativity, resilience, and vision. It calls for embracing innovative approaches like decentralized AI, where flexibility, openness, and a shared sense of responsibility guide the course of development. As we navigate the challenges and uncertainties that lie ahead, let us keep in mind that just as nature finds its way in the face of adversity, so too can we find our path in the evolving landscape of AI. Embracing decentralization is not merely an alternative strategy; it’s an affirmation of our ability to adapt, innovate, and guide the progression of AI for the benefit of all. Evolution always finds a way; let’s ensure our technological evolution does so with a commitment to transparency, inclusivity, and the common good.
References
- D, Orfeas. (2023, April 5). Making Anarchist LLM. Doxometrist. https://doxometrist.substack.com/p/making-anarchist-llm
- Cheung, E. (2023, April 21). Chinese quantum computer is 180 million times faster on AI-related tasks, says team led by physicist Pan Jianwei. South China Morning Post. https://www.scmp.com/news/china/science/article/3223364/chinese-quantum-computer-180-million-times-faster-ai-related-tasks-says-team-led-physicist-pan
- OpenAI. (2021). ChatGPT. https://www.openai.com/research/chatgpt
- GRIDNET. (2017-2023). GRIDNET Decentralized Operating System. MDPI Research Paper
- Shen, S., Church, G., & Zhang, F. (2023). The Pile: An 800GB Dataset of Diverse Text for Language Modeling. https://arxiv.org/abs/2101.00027
- Cerebras Systems. (2023). The World’s Fastest AI Computer. https://www.cerebras.net
- OpenAI. (2021). GPT-4. https://www.openai.com/gpt-4
- Quantum Computing Report. (2023). Quantum Computing. https://quantumcomputingreport.com
- Jiuzhang Quantum. (2023). Jiuzhang Quantum Computer. https://www.jiuzhangquantum.cn
- Network Science. (2023). Network Analysis. https://www.networkscienceinstitute.org
- Satoshi, Nakamoto. (2008). Bitcoin: A Peer-to-Peer Electronic Cash System. https://bitcoin.org/bitcoin.pdf